“Data Analysis and Econometrics” - course 34,000 rubles. from MSU, training 12 weeks. (3 months), Date: November 29, 2023.
Miscellaneous / / December 01, 2023
The main goal is to introduce students to the methods of econometric analysis used in business and modern research. The program will help you better understand how to apply econometric methods in solving applied problems in business, what they write in scientific articles, as well as carry out their own econometric research.
The main goal is to introduce students to the methods of econometric analysis used in business and modern research.
The program will help you better understand how to apply econometric methods in solving applied problems in business, what they write in scientific articles, as well as carry out their own econometric research.
Who is this program for:
For everyone who is faced with the need to identify cause-and-effect relationships and make forecasts based on statistical data
Does not require strict mathematical preparation requirements. Knowledge of the basics of probability theory and mathematical statistics will be useful, but not required.
What will mastering this program give you:
Learn to collect and prepare information, as well as do preliminary data analysis;
Learn how to formulate economic hypotheses in terms of econometric models;
You will be able to carry out econometric calculations using econometric software to test your hypotheses regarding the analyzed data
You will be able to evaluate the quality of the resulting econometric models;
Be able to correctly interpret the results of econometric modeling
Documents upon completion of the program: Certificate of advanced training
Duration
3 months, 72 hours
Form of study: correspondence using distance technologies
Introduction
You will learn what econometrics is and why it is needed. Review the applications of econometrics in applied research and examples of questions that can be answered using it. Learn what types of data are used in econometric modeling.
They will tell you what is: paired regression, derivation of formulas for estimating coefficients in paired regression, R-squared coefficient, asymptotic properties of OLS estimates, prerequisites for a linear model of paired regressions, testing the statistical significance of coefficients, confidence intervals, homoscedasticity and heteroskedasticity, standard conditions consistent with heteroscedasticity errors
2 Multiple regression
Motivation for using multiple regression. Assumptions of a linear multiple regression model. Testing hypotheses and constructing confidence intervals.
3 Multicollinearity. Dummy variables
Multicollinearity. Dummy (binary variables) shift and slope.
Transforming variables into regression models. Linear, logarithmic, semi-logarithmic and other forms of dependence. Meaningful interpretation of coefficients. Recommendations for the presentation of econometric research results.
4 Regression equation specification
Endogeneity. Consequences of regression model misspecification. Substitute variables. Criteria for deciding whether to include a variable in the model. Specification tests.
5 Instrumental variables
Implications of correlated explanatory variables and random errors. The problem of endogeneity. Instrumental variables. Two-step least squares method.
6 Panel data models
Advantages of models using panel data. Simple full (pooled) regression, fixed effects model, random effects model. Model type selection test.
7 Binary choice models
Linear probability model (LPM). Advantages and disadvantages of LVM. Logit model, probit model. Estimation of parameters of logit and probit models. Interpretation of coefficients in logit and probit models (calculation of marginal effects). Estimation of the quality of logit and probit models. Testing the significance of coefficients in logit and probit models.
8 Forecasting from time series data
Time series. Definitions and examples. Stationarity and non-stationarity. Unit roots. Processes AR(p), MA(q), ARMA(p, q). Random walk. Process integrated of order k. ARIMA(p, k, q) process.
Unit root testing.
Evaluating ARIMA models. Model identification procedure. Forecasting in ARIMA models.
Autoregressive conditional heteroscedasticity (ARCH) model. Various generalizations of the autoregressive conditional heteroskedasticity model (GARCH and others). Estimation and forecasting.
Autoregressive distributed lag models. Estimation and forecasting.
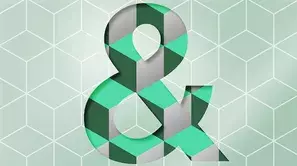
The course introduces students to mathematical logic, its methods, theorems, and applications. In the process of studying the course, students will be able to learn about various logical systems - classical logic, intuitionistic logic, various modal logics, as well as classical predicate logic and theories constructed based on it.
4,2
for free